Mistakes from my Failed Startup in Scalping Concert Tickets
And why you will never get Taylor Swift tickets at face value
This is part of an ongoing series of essays to deconstruct the past startups that I’ve worked at and what separates successful companies from failures? Part 1 went over how the first startup I joined Jobr got acquired for millions in two years.
In November 2022, Taylor Swift fans crashed Ticketmaster. Not just the website - they crashed the entire company's facade of competence. Over 14 million people tried to buy tickets meant for 1.5 million fans. The servers melted down, the presale collapsed, and Ticketmaster had to cancel the general public sale entirely. Swift herself said watching her fans struggle to get tickets was "excruciating."
The drama was so bad it triggered a Senate hearing. Ticketmaster executives were hauled in front of Congress to explain how they could fumble the biggest tour launch in history. But while everyone was focused on the technical failure, they missed the most interesting story: Those $100 "face value" nosebleed tickets were selling for $1000, $2000, even $5000 on resale markets.
The concert industry has a dirty secret. It's not just that Ticketmaster is evil, or that bots are stealing tickets. It's that the entire market is built on a comfortable lie: Artists pretend their tickets are affordable, Ticketmaster pretends they're fighting scalpers while giving kickbacks to artists, and fans pretend they won't pay whatever it takes to get in.
But there's a fourth player in this game. One that nobody likes to talk about, yet somehow ends up with 95% of the tickets meant for fans. The profiteers, the bots, the scalpers, the ticketing resellers - shadow players operating in this space who quietly make millions of dollars per year while everyone else points fingers at Ticketmaster.
And in early 2017, years before the Swift meltdown, I wanted to be one of them.
Building a Model
Six months after the acquisition by Monster, I was getting bored. I was in peak concert attending stage-of-life as a 20 something with disposable income. And after getting stiffed from a few sold out concerts, I naturally changed my attention to, “Wait, how do I use my data science skills to make money from this instead?”
My core thesis was simple but powerful: If I could ingest enough data from Ticketmaster, Stubhub, and Spotify, I could train a model to predict the "true price" of concert tickets. Not the price artists claimed they were worth - the price people would actually pay. And Ticketmaster themselves made the best case for how it all worked:
Artists underprice tickets for several reasons, but mostly out of regard for their fans. There is a kind of social compact between performers and their devoted fans to charge them fair prices, not whatever the market will bear. StubHub and the resale marketplaces that followed it changed that forever by presenting artists with 24/7 reminders of what the true market value for their tickets is — and that when they don’t charge those prices scalpers will find ways to acquire tickets and resell them at full market value
Translation: The entire industry runs on artificial pricing. Just like hedge fund managers provide liquidity in stock markets, resellers were actually providing price discovery in a market built on lies. You could argue the resellers weren’t the bad guys - they were just revealing what the tickets were actually worth.
For someone like Taylor Swift, the math was brutal: Those $100 face-value tickets were selling for 10 to 50x their price on secondary markets. Either she truly believed her tickets were only worth $100 (unlikely), or she was deliberately underpricing to maintain her image as an artist who cares about fan access.
But here was the edge: For 95% of touring artists, predicting the true resale price required data that normal fans didn't have access to. If I could build a model accurate enough to spot underpriced tickets across the U.S., I'd have a money-printing operation on my hands.
The engineering challenge was enormous. I needed to build:
A data ingestion pipeline for every new concert announcement and sale time
Daily scrapers to track pricing trends across StubHub and other resale sites
Systems to pull artist popularity metrics from Spotify, Google Trends, and social media
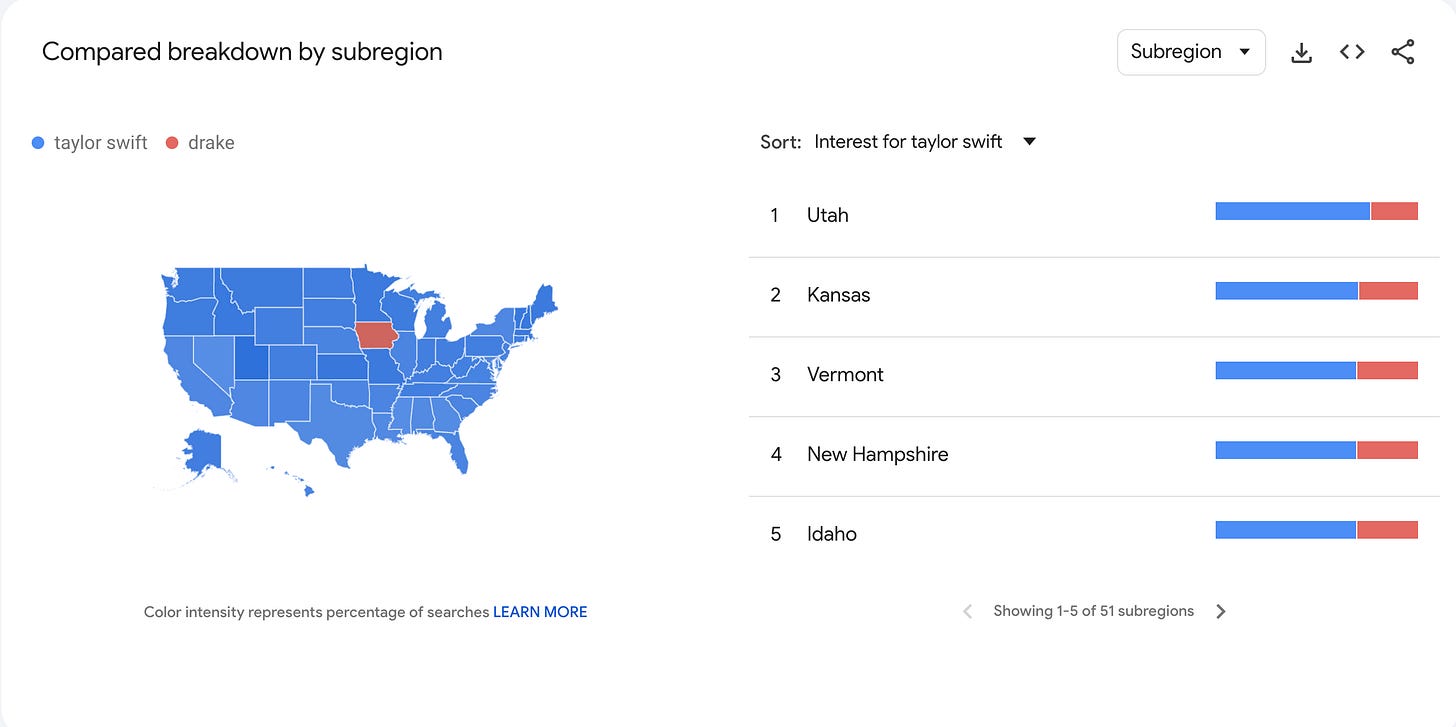
And that made it sound simple. Just getting tour dates was a nightmare - every venue used different ticketing vendors like Ticketmaster, AXS, or Ticketfly. Each had their own APIs (or lack thereof) and anti-scraping measures. I'd need to bypass them all just to get basic concert metadata.
I quickly realized I needed at least a year of historical pricing data to build anything accurate. But I was too impatient to spend a year only just coding. So I decided to start small: I'd buy and flip tickets manually, learning the industry's unwritten rules while gathering data for my eventual automated system.
I had no idea what I was getting myself into.
The Beginning of the Problems
My worst losses came early, when I naively assumed that "popular artist = easy profit." The reality was far more complex. While manually flipping tickets, I started noticing patterns that every experienced scalper already knew:
One was that day of the week was everything. Thursday through Saturday shows were gold mines; Sunday through Wednesday were risky bets.
Hometown shows for any artists? Guaranteed sellouts. Old band that was touring again? Old people had money.
First tours after a breakout album? Pure profit, their agents would book them into venues way too small, playing it safe before the artist blew up. And when Harry Styles or Drake dropped a bigger album than their last? You could bet those tickets would vanish instantly.
But for every obvious win, there was a brutal lesson. I lost big on Katy Perry's comeback tour (turns out no one wanted that comeback). I completely whiffed on LCD Soundsystem playing four nights in NYC - a city with seemingly unlimited demand for indie rock legends.
And as I worked on my pricing model, I realized I needed to build two distinct models:
Predicting whether a specific concert would sell out in any given city
Predicting what tickets would actually sell for on the secondary market at any point between announcement and showtime.
The chaos of the industry made both predictions nightmarish. Artists would sell out a show, watch resale prices skyrocket, then announce a second night - instantly nuking the value of every resold ticket from the first show. I had to constantly monitor venue schedules, artist popularity metrics, and a dozen other factors just to avoid getting burned.
But the real problem? Even when I knew a show would sell out, I couldn't get the tickets. Ticketmaster's anti-bot measures worked frighteningly well against amateurs like me, while professional scalpers had figured out how to bypass them entirely. Even basic VPN usage was a nightmare, Ticketmaster would blacklist entire VPN services and shadowban their IPs. You'd think you were about to score tickets, watching that loading spinner go round and round, but the system was just ghosting you, never intending to complete the sale.
To compete with the pros, I'd need to engineer an entire system that could coordinate multiple accounts, rotate through fresh IP addresses, and somehow solve CAPTCHAs at lightning speed.
The technology required was far more sophisticated than I'd imagined. As one industry insider explained:
“The technology pings the on-sale while a room full of people, usually off-shore in a second or third world country, furiously bang out the re-captcha with no idea of what they’re actually doing. The operator sits back and watches all the options populate on their screen and chooses which tickets to buy. So not only are they getting tickets fans can’t get and blocking others from buying them, they are cherry picking the best tickets and passing on the less desirable, releasing them back into the on-sale for the “lucky” fan who gets them.”
The economics were brutal too. Stubhub would take 30% in total fees: 20% from buyers and 10% from sellers. So when I guessed wrong about demand, I'd take massive losses. I once had to sell Feist tickets in NYC for $6 that I'd bought for $60, just to get something back after fees.
Then there was the logistics nightmare. Ticketmaster started mailing physical tickets for high-demand shows to prevent resale. I once had to beg my roommate to ship overnight tickets to a buyer while I was vacationing in Iceland. Needless to say, she did not do it.
Giving Up
After six months of buying and reselling tickets, I wasn't even sure if I'd broken even. The model I'd built on a few hundred data points couldn't predict better than my gut instincts, and it was becoming clear that professional brokers were operating on a level I couldn't comprehend.
Then I stumbled onto something interesting. While developing my data ingestion system, I discovered an open Ticketmaster endpoint that revealed the exact number of tickets remaining for any show. With enough data points, I could build a time forecasting model to predict when concerts were about to sell out.
It felt like striking gold. Instead of fighting bots and insiders for Taylor Swift tickets, I could find arbitrage opportunities across the vast landscape of shows that didn't sell out immediately. My strategy was simple: buy tickets when a show hit 95% capacity, effectively cornering the market on remaining inventory, then flip them on StubHub.
But the market had other plans. Shows that sold out last-minute often lacked enough buyer demand to move the tickets profitably. Many concerts never sold out at all. And when my strategy did work, StubHub's fees ate so much of the margin that it wasn't worth scaling.
Eventually, the moral weight of scalping started to gnaw at me. I tried pivoting to a more ethical business model - something like Scott's Cheap Flights, but for concert tickets (I called it Jay’s Cheap Tickets). I'd use Spotify integration to alert fans when to buy tickets for their favorite artists. But without frontend engineering skills, that dream died after an ugly MVP.
Competing against Ticket Brokers
What I didn't understand then was the true scale of the ticket broker ecosystem. At the highest level, these weren't just scalpers - they were sophisticated operations with insider connections. As one industry source explained:
“Brokers offer these insiders a way to profit from their tickets. An untraceable way of selling their tickets for a profit without being caught. And since the insider themselves can't post or sell the ticket for market value, they will usually sell to the broker for less than market, allowing the broker to make a profit on the deal.”
Some brokers established formal "sponsorship" agreements for bulk purchases. Others bought season tickets across multiple teams, using premium games to subsidize losses on less popular events.
The reality of this world hit home when a friend texted me about a party in San Diego, hosted in a “mansion of a house” by the CEO of Swagg Seats. Their website reveals almost nothing about their operation, just a 2020 video initially talking about promoting "positivity and progress" and a mission "to allow anyone to get a seat into their favorite concert."
But then he also explains how he makes $20 million a year from ticket scalping:
We’re buying all day long. These are people who commit 40-50 hours a week to getting the best seats in the house. Not only are they knowledgeable on how to do that, but they have access to market data that's coming in from multiple sources.
Here's how it works: let's use the example of the Eagles announcing their Hotel California album tour. Ticket brokers immediately list speculative tickets for sale on major ticketing websites like StubHub before the first presale even takes place on Ticketmaster. Swagg Seats is able to access market data from each city to see which event dates would be the most popular and what locations to buy to maximize profits.
The growth was staggering. And he continued to boast:
The business is very profitable as it is right now. Swag Seats, for the first five or six years, was a one to two million dollar company, and we were able to grow in the last three or four years to 20-plus million. It's come a long way, that’s for sure.
I started off with myself and a laptop right on Mission Beach, you know, sitting on top of the boardwalk buying lawn seats to an amphitheater show. Now, on any given morning, we might buy close to a million dollars’ worth of tickets for a variety of tours. We want to grow, double, and triple each year in terms of being able to sell more. If you're investing in Swag Seats, you're investing in an established commodity. We've already pushed eight figures this year; we're very, very profitable already.
Here was my counterfactual, the shadow ticket broker who'd solved all the market liquidity problems I'd struggled with. Swagg Seats combined advanced data analytics, insider partnerships, and every possible edge from fan club memberships to credit card deals to build a scalping empire.
But there was a dark irony: while they claimed to be getting tickets to fans who wanted them, they were actually creating artificial demand and constraining supply through their market power. And by 2024, the industry has seemingly shifted so dramatically that some artists are commonly facing the opposite problem - struggling to sell out shows at all.
Conclusion
In November 2023, as I watched another Taylor Swift ticket crisis unfold - this time for her Eras Tour movie - I couldn't help but laugh. Here we were again: crashed servers, angry fans, and surprisingly, a few texts from people who knew I worked on the concert ticketing project. Their ask? If I had snatched up any extra Taylor Swift tickets.
My failed attempt at this startup taught me a few crucial lessons:
First, I drastically underestimated the scope of what I was building. I thought this was a data science problem: write some clever algorithms, predict some prices, profit. But real arbitrage operations aren't just about spotting opportunities; they're about building infrastructure to capture them at scale.
This hit home when I read Michael Lewis's "Going Infinite" about Sam Bankman-Fried's initial fortune. Before the fraud and collapse, SBF made his first millions through legitimate Bitcoin arbitrage - buying BTC in Korea for $18K and selling it in the U.S. for $20K. The price difference was obvious to anyone looking at the markets. The hard part? Building a system to actually capture it: opening banks in multiple countries, managing currency transfers, navigating regulations. Just like my ticketing flipping dream, seeing the opportunity was the easy part.
Second, "motivation half-life" is real. I attempted this project three different times, each with diminishing enthusiasm. The final death knell came when I stopped paying Google Cloud Platform bills for millions of data points I'd collected. It wasn’t worth the hosting bill to just store it on RDS.
But the experience wasn't worthless. I proved to myself I could build serious data engineering infrastructure and gave me drive to do something outside of my day job. I just didn’t know what a successful one looked like just yet.
Fascinating, thanks for sharing!
Great piece –I learned a lot.
Super minor correction: BTC wasn’t priced cheaper in Korea; it was priced higher. The actual arbitrage profits depending on the price differential of XRP: so you are right that Alameda was buying BTC in Korea and selling in the US, but they actually LOST money on that trade; they made their money because they were selling XRP in Korea at an even larger profit (than what they lost on BTC). The numbers are on p.93 of Lewis’s book, and I’ve spelled out all the details of the arbitrage in a Substack piece of mine, here: https://moneypower.substack.com/p/how-to-tell-the-story-of-crypto-part-5e7